The Kohl Centre Workshop
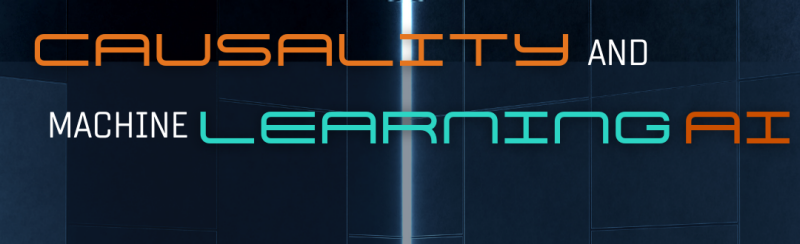
Thank you to everyone who joined us for this workshop. It was a fantastic turnout. A special thanks to our presenters and sponsors. You may view the event photos here.
I welcome any feedback or other engagement from the presenters, sponsors, and participants.
Le Wang, Professor and David M. Kohl Chair
Scholars from renowned universities such as Cornell, University of Chicago, and UC Irvine, along with various departments at Virginia Tech, are coming together to explore the critical intersections of causality and machine learning/AI.
Causality is pivotal in social science, business, and applied economics, shaping the future of machine learning and AI applications in diverse industries. This fundamental concept has earned prestigious recognition, with the 2021 Nobel Prize in Economics and the 2012 Turing Award in Computer Science honoring contributions to causality.
The upcoming workshop aims to foster collaborations that transcend traditional boundaries, drive research forward, and align closely with Virginia Tech's cutting-edge work.
This workshop is in partnership with and sponsored by:
The details
Pre-workshop: Tutorial session on causal machine learning by Hengrui Cai, author of Causal Decision Making
- Date: Friday, November 1, 2024
- Time: 2:00 - 5:00 pm
- Location: Room 132 in the Data and Decision Sciences Building
Workshop:
- Date: Saturday, November 2, 2024
- Time: 8:30 am - 5:00 pm
- Location: The Inn at Virginia Tech and Skelton Conference Center; Solitude Room
8:30 - 9:00 am | Meet and greet over coffee and snacks
9:00 - 9:15 am | Welcome
- Dan Sui
Senior Vice President and Chief Research and Innovation Officer - George Davis
Interim Department Head, Department of Agricultural and Applied Economics - Le Wang
Director of the Kohl Centre and David M. Kohl Chair and Professor in the Department of Agricultural and Applied Economics
9:15 - 10:30 am | Session 1
- Lydia Manikonda
Title: Causality in the Age of Generative AI - Hengrui Cai
Title: Towards Trustworthy Machine Learning: A Causal Lens on Learning Non-Spuriousness - Jacek Kibilda
Title: Current and Prospective Uses of AI/ML in Addressing Problems Related to 6G Security
10:30 - 11:00 am | Coffee and networking break
11:00 am - 12:15 pm | Session 2
- Rui Fan
Title: Rehabilitating the Once-Abandoned Endogenous IV - Zhenshan Chen
Title: Causal Machine Learning for Policy Evaluation with Housing Transaction Data - Kyle Butts
Title: Dynamic Treatment Effect Estimation with Interactive Fixed Effects and Short Panels
12:15 - 1:30 pm | Lunch - Smithfield Room
If you are interested in one of the available lunch spots (limited to 30 participants), please email Jennifer Shelton. Spots will be allocated on a first-come, first-served basis.
1:30 - 3:10 pm | Session 3
- Wenzhuo Zhou
Title: Bi-Level Offline Reinforcement Learning - Klaus Moeltner
Title: Controlling for problematic responses in survey data: A Causal Forest approach - Bo Zhou
Title: Valid Post-Inference for Contextual Bandit Problems - Xinran Li
Title: Randomization inference and sensitivity analysis for quantiles of individual treatment effects
3:10 - 3:30 pm | Coffee and networking break
3:30 - 5:00 pm | Session 4
- Louise Laage
Title: Estimating Stochastic Block Models in the Presence of Covariates - Yan Xu
Title: Heterogeneous Complementarity and Team Design - Christina Yu
Title: Causal Inference in the Presence of Network Interference with Low-Order Interactions
Contact: Le Wang
Director, Kohl Centre and David M. Kohl Chair and Professor in the Department of Agricultural and Applied Economics
Assistant professor of economics at the University of Arkansas: Webpage >>
Assistant Professor in Statistics in the Donald Bren School of Information and Computer Sciences at the University of California Irvine: Webpage >>
Assistant Professor in the Dept. of Agricultural and Applied Economics at Virginia Tech: Webpage >>
Assistant Professor of Economics with Rensselaer Polytechnic Institute: Webpage >>
Research Associate Professor with the Commonwealth Cyber Initiative and Bradley Department of Electrical and Computer Engineering at Virginia Tech: Webpage >>
Assistant Professor in the Department of Economics of Georgetown University: Webpage >>
Assistant Professor in the Lally School of Management at Rensselaer Polytechnic Institute: Webpage >>
Professor in the Dept. of Agricultural and Applied Economics at Virginia Tech: Webpage >>
Assistant Professor at Cornell University in the School of Operations Research and Information Engineering: Webpage >>
Assistant Professor of Marketing in the Pamplin College of Business at Virginia Tech: Webpage >>
Assistant Professor of Economics in the Dept. of Economics at Virginia Tech: Webpage >>
Assistant Professor in the Donald Bren School of Information & Computer Sciences at the University of California Irvine: Webpage >>